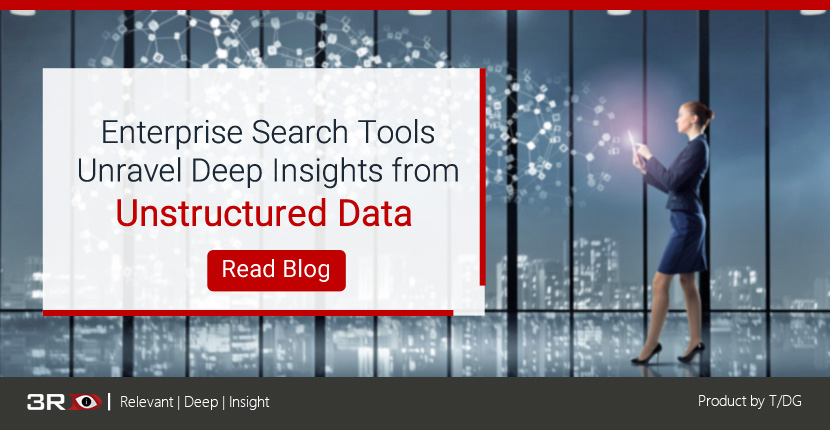
Structuring Unstructured Data with Enterprise Search
Picture this:
- According to Big Data Made Simple, the volume of data created by U.S. companies alone each year is enough to fill 10000 Libraries of Congress… and
- Most companies only analyze 12% of the data they have!
What is the scenario depicted by these two stats you see here? Well, these statistics say, that in spite of the fact that data is growing in unsurmountable volumes, a lion's share of enterprises still do not have the means to analyze the large volumes of data that they have.
So, that makes one wonder – why does such large volumes of data simply don't get analyzed? It's because it mainly consists of unstructured data, as depicted by the following stat –
- According to a Gartner report, close to 80% of the world's data is unstructured.
What is Unstructured Data
So, what is unstructured data and why do enterprises find it so difficult to analyze unstructured data?
Wikipedia defines unstructured data as "information that either does not have a pre-defined data model or is not organized in a pre-defined manner". It further adds that "Unstructured information is typically text-heavy, but may contain data such as dates, numbers, and facts as well. This results in irregularities and ambiguities that make it difficult to understand using traditional programs as compared to data stored in fielded form in databases or annotated (semantically tagged) in documents". The unstructured data in enterprises consists of data from varied sources and in diverse formats, such as social media posts, emails, word documents, photos, videos, webpages, audio files, and more.
Unstructured data is fundamentally different from structured data, because while structured data is generated by computers, unstructured data is generated by humans. So, while the latter is formatted and organized, the former is inconsistent but unique. The unorganized nature of unstructured data
However, even though unstructured data is difficult to analyze, the fact is that the unstructured data that enterprises have, holds deep insights hidden within. These insights, if unraveled, can help a business formulate strategic business decisions to fuel business growth.
The Need for Structuring Unstructured Data
Deriving insights from data involves analyzing the data to unravel trends, facts and statistics and presenting them in graphical form.
A new age enterprise search tool like 3RDi Search is what enterprises need to analyze unstructured data successfully. However, before the large volumes of unstructured data can be analyzed to derive insights from it, the first step is to convert it into a structured format. This step is also the most important step in the entire process of text mining because the efficiency with which unstructured data is converted to structured data goes on to determine the efficiency of the indexing process, which in turn affects the accuracy of data and the insights thus derived.
The Process of Deriving Insights from Data
The process of deriving key insights from data involves the following steps:
1] Data Acquisition: Data acquisition refers to the conversion of data that is available in various units of measurement, into a format that is easily understood by computers. In other words, it is the process of making data accessible to computers for processing and analysis.
2] Data Cleansing: Data cleansing refers to the process of detection and removal of inaccurate and/or corrupt fragments of data from a data set. It's a process of identifying the details withing the data set that are duplicate, irrelevant or incomplete and removing them, so that the data set is ready to be analyzed.
3] Data Enrichment: Data enrichment is the process of merging the data derived from reliable external sources for the purpose of making the existing data more useful and enriched. Data from social media is a good example of data that is merged with enterprise data to help decision makers with useful insights about the market trends and the habits of their users.
4] Text Mining: Text mining is the process of extracting high quality insights from text. It involves a lot of processes and the key processes involved in text mining are as follows:
- According to a Gartner report, close to 80% of the world's data is unstructured.
- Statistical pattern learning
- Data parsing
- Text categorization
- Text Clustering
- Entity extraction
- Creation of taxonomies
- Content summarization
- Sentiment analysis
- Entity relation modelling
5] Natural Language Processing: As we have learnt earlier, unstructured data is data that is generated by human beings and so Natural Language Processing (NLP) is a technology that is immensely useful in the process of text mining. NLP turns the text into data that can be understood and analyzed by the enterprise search engine.
6] Data Quality Analysis: The last step in the process of deriving insights from the data involves sampling the data to ensure all the above processes have been accurately and successfully incorporated.
The Final Word:
The analysis of unstructured data is a very complex process that involves the use of advanced technology like natural language processing, machine learning and semantics. Since a large portion of enterprise data is unstructured, enterprises today are investing in the new age enterprise search tools to help them derive deep insights from unstructured data.